AI is sleepwalking us into surveillance
The harsh reality of AI’s unconsented data collection
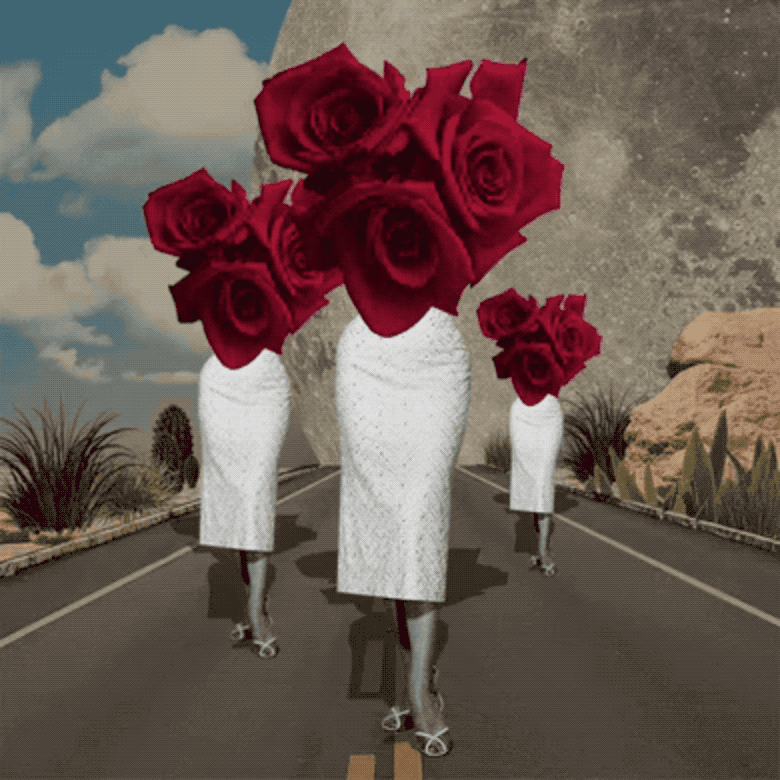
In 2020, a photograph of a woman sitting on a toilet was leaked on the internet, which sparked a huge controversy. The Roomba J7 robotic vacuum cleaner took this picture as part of its routine data collection. This was just one among a series of pictures that captured entire households, objects and the people living in them. A group of gig workers from Venezuela contracted to label images for training AI models, received these pictures which were later leaked online.


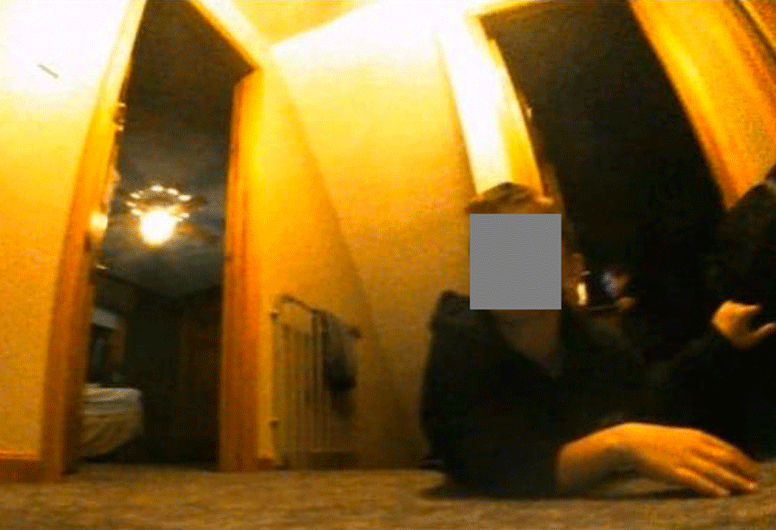
In the race to make each new AI model better than the previous one, companies are resorting to unethical data collection techniques to stand ahead in the competition. Our private data, including medical records, photographs, social media content, etc., are all making their way into data sets for training AI models.
Your data is being stolen
Are you willing to give up your privacy for convenience?
Reading all the privacy policies you encounter in a year from big tech companies would take 30 full working days of your life.
Source: The cost of reading privacy policies
Home is our safe space, but what happens when the appliances in our household start to leak our data? Kashmir Hill and Surya Mattu, investigative data journalists, revealed how the smart devices in our homes are doing precisely this. At first, it might sound mundane that your electric toothbrush is routinely sending data to its parent company. However, they reveal in their 2018 TED talk how some of this collected data can come back to haunt us. For example, your dental insurance provider can buy your data from the toothbrush company and charge a higher premium if you miss brushing your teeth at night.
Data sets used for training image synthesis AIs are made by scraping images on the internet, which copyright holders and subjects may or may not have given their permission to be used. Even private medical records of patients end up as training data for AI models. Lapine, an artist from California, discovered that her medical record photos taken by her doctor in 2013 were included in the LAION-5B image set, a data set used by Stable Diffusion and Google Imagen. She discovered this through the tool: Have I Been Trained, a project by artist Holly Herndon that allows anyone to check whether their pictures have been used to train AI models.
The LAION-5B dataset, which has more than 5 billion images, includes photoshopped celebrity porn, hacked and stolen nonconsensual porn, and graphic images of ISIS beheadings. More mundanely, they include living artists’ artwork, photographers’ photos, medical imagery, and photos of people who presumably did not believe that their images would suddenly end up as the basis to be trained by an AI.
Source: AI Is Probably Using Your Images and It’s Not Easy to Opt Out, Vice
Compromised identities
Research proves that ai-generated faces can be reverse-engineered to expose actual humans that inspired it

AI-generated faces are mainstream now; designers are using them as models for their product shoots or in fake personas. The idea was that since these were not real people, they wouldn’t need consent. However, these generated faces are not as unique as they seem. In 2021, researchers were able to take a GAN-generated face and backtrack it to the original human faces from the dataset that inspired it. The generated faces resemble the original ones with minor changes, thus exposing the actual identities of people in these datasets.

Unlike GANs, which generate images that closely resemble the training samples like above, diffusion models (DALL-E or Midjourney) are thought to produce more realistic images that differ significantly from those in the training set. By generating novel images, they offered a way to preserve the privacy of people in the dataset. However, a paper titled Extracting Training Data from Diffusion Models shows how diffusion models memorize individual images from their training data and regenerate them at runtime. The popular notion of how AI models are “black boxes” which reveal nothing inside is being revisited through these experiments.
AI surveillance
Supercharging the surveillance society with AI
One of the most controversial cases of intelligent surveillance occurred during the Hong Kong protests in 2019. The police used facial recognition technology to identify the protestors and penalize them individually. The protestors realized this and aimed their hacked laser pointers at the cameras to burn their image sensors.


Half of all the surveillance cameras in the world are in China, making them the world’s largest surveillance society. They use it for fining jaywalkers or for airport security checks using facial recognition. While AI-based surveillance systems may seem like a valuable tool in the fight against crime and terrorism, it raises concerns about privacy and civil liberties. These systems point towards an Orwellian future where Big Brother can monitor and control individuals, potentially losing freedom and civil liberties.
Who’s fighting back?
The US Federal Trade Commission (FTC) has a provocative answer to privacy-infringing AIs; they have begun practicing algorithmic destruction. They demand that companies and organizations destroy the algorithms or AI models that it has built using personal information and data collected in bad faith or illegally. Below are a few other examples of initiatives:
Policies and frameworks
The European Union (EU) Parliament has taken a significant step towards protecting individual privacy in the age of AI. They have backed the proposal to ban AI surveillance in public spaces. EU’s widely popular General Data Protection Regulation (GDPR) also has the best data protection framework, which requires consent from individuals before collecting and using their data.
Canada’s Personal Information Protection and Electronic Documents Act (PIPEDA) requires businesses to maintain “reasonable” security safeguards to protect personal information. And in the US, the California Consumer Privacy Act (CCPA) is a comprehensive privacy law granting individuals the right to ask what personal information organizations have collected, how it is used, and for what purpose.
Projects and experiments
- Have I Been Trained: A platform that makes it easy to search whether or not our data has been used to train AI. Upload your photos to check if it has made their way into the datasets of diffusion models. This is part of a bigger project called Spawning.ai, which lets people take back control of their data. They are also developing opt-out and opt-in tools that enable you to decide whether your data should be used for training.
- Microsoft Synthetic Faces: A set of 100,000 synthetic faces from Microsoft that can be used to train face recognition algorithms without using real people.
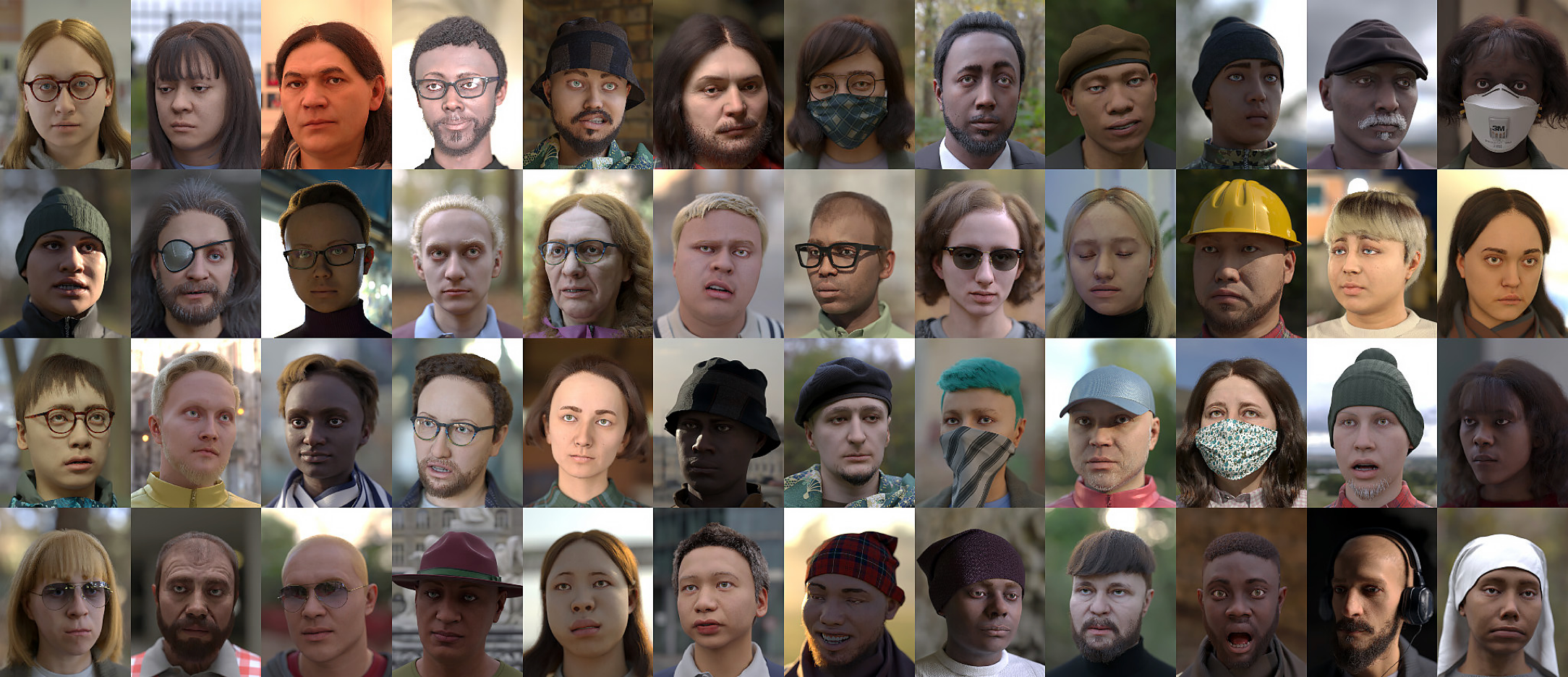
- AI Camouflage: Adversarial patches which can be embedded into clothes and other objects that confuse AI classification systems.
- Adversarial Makeup: Face makeup that confuses AI classification systems and makes you invisible. CV dazzle also offers Youtube makeup tutorials:
- Alias: A privacy intervention from Bjørn Karmann that blocks voice assistants from listening to you all the time.

In his book: New Dark Age, James Bridle makes a commentary on how AI surveillance is turning clinical paranoia into a reality. “One of the first symptoms of clinical paranoia is the belief that somebody is watching you; but this belief is now a reasonable one. Every email we send; every text message we write; every phone call we make; every journey we take; each step, breath, dream, and utterance is the target of vast systems of automated intelligence gathering, the sorting algorithms of social networks and spam factories, and the sleepless gaze of our own smartphones and connected devices. So who’s paranoid now?”
*For further resources on this topic and others, check out this handbook on AI’s unintended consequences. This article is Chapter 3 of a four-part series that surveys the unintended consequences of AI.